In the first part of this report, we considered how the move to cloud-based resources for data storage, application delivery and infrastructure management is coinciding with the proliferation of new, smarter gadgets and monitoring devices – and the need to make sense of all the data that they produce.
Learning systems based on self-educating algorithms are both contributing to this data pool, and providing new opportunities for coping with the challenges and insights that derive from it.
In this concluding part, we’ll look at how emerging trends and the current crop of machine learning-based analytics tools are combining toward a possible future where self-educating networks may be able to analyse and manage their own infrastructure.
Infrastructure Analytics as an Ideal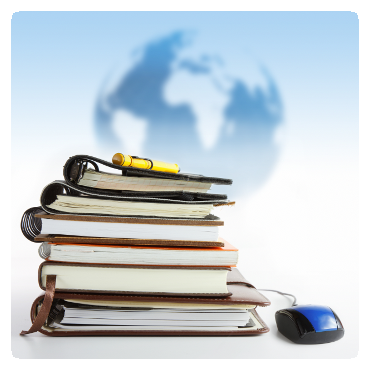
For many enterprises, IT infrastructure is no longer a homogeneous landscape of desktop computers, file servers, and associated peripherals. Laptops, tablets, smartphones, and a host of other wearable or mobile devices may figure, as Bring Your Own Device or BYOD policies allow workers to add hardware from a disparate range of manufacturers to the mix.
A partial or total dependence on cloud-based resources often comes into play, as data and applications are ported through private or hybrid clouds, virtual desktop environments, hosted VoIP communications systems, and hyper-converged infrastructures. The increasing prevalence of devices and commodities from the Internet of Things (IoT) adds to the overall complexity of many of today’s systems.
With such a heterogeneous mix of hardware and software elements, managing the new breed of corporate infrastructures could be a nightmare task, imposing huge demands on administrators.
But what if the burden of monitoring and allocating resources, troubleshooting problems, and reporting on real-time performance could be shifted (at least in part) to the network, itself? With the incorporation of machine learning technologies into a network’s infrastructure, it could be possible to:
· Get an overview of complex networks in real time, that can watch for unanticipated patterns of resource consumption, adjust their allocation to deal with peaks, lulls, or emergencies, and anticipate future demands.
· Determine the cause of infrastructure events as they happen, and respond instantaneously.
· Create a responsive learning environment that can extrapolate from observed events to predict future outcomes, and intervene right now, to prevent harmful ones from happening.
Infrastructure Analytics as a Practical Reality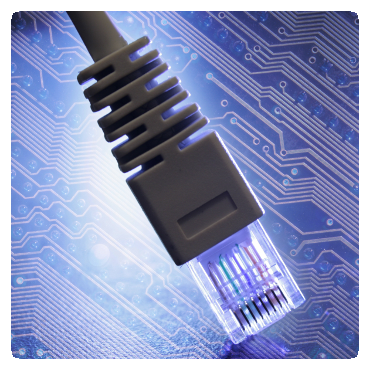
There are several conditions that will have to be met, before this idealised model of self-educating infrastructure management can become a reality.
First, an intelligent management system will be called upon to continuously monitor the behaviour of its host system, and adjust the allocation of network resources to respond to changes in real time. Note that with a mix of hardware and software from different vendors, as well as cloud hosting services (which may also be sourced from a range of third parties), the onus will be on a learning infrastructure to shift workloads and allocate resources across multiple manufacturers, as and when required.
Secondly, machine learning capabilities will have to be deployed to allow an infrastructure analytics system to create logical associations between the observed causes and effects of various network events. This may provide the basis for governing its response to known triggers.
Going beyond this, a self-educating system should be able to create simulations and models to test out various infrastructure performance factors under varying conditions. These will become the basis for predictive analysis and pre-emptive action. Moreover, the results of these simulations should be presentable in an easy to read format, using dedicated reporting tools and user-friendly dashboards.
Finally, intelligence in infrastructure analytics should be a boost to the overall resilience of a network. Beyond its capability to predict failures of components and processes and take action as problems occur, the system should be able to suggest methods of preventing those failures from happening, through performance tweaks that make failure less likely, and ultimately through the introduction of elements or routines that preclude the possibility of failure, altogether.
Machine Learning as a Service
We’re already part of the way there. Several leading vendors are currently offering “Machine Learning as a Service” or MLaaS platforms, which incorporate a spectrum of existing technologies ranging from speech and facial recognition to natural language processing, deep neural networks (a.k.a. deep learning), and predictive analytics.
MLaaS Platforms
Watson Analytics from IBM uses a natural language interface to deliver data visualisations and predictive analytics. Its free trial version imposes limits on the amount of data that can be analysed.
Visualisation tools and software wizards are key to the Amazon Machine Learning service, which guides developers through the process of creating machine learning models. It uses simple APIs (Application Programming Interfaces) and Amazon’s cloud-based infrastructure management to enable subscribers to get predictions from their applications, without having to generate their own custom code.
Medium and large-scale enterprises may appreciate the low pricing and relative simplicity of BigML, a cloud-based machine learning platform that uses the REST API to generate production-grade applications with predictive capabilities. Predictive models may be built using Supervised Learning, while behavioural analysis may be conducted via Unsupervised Learning. Tools for Anomaly Detection and data visualisation may also be incorporated.
These are just a few examples in a market that includes offerings from the likes of Microsoft, Google, and a range of third-party service providers.
Current and Future Trends
We’re still in the early stages of an evolving technology landscape that’s seen machine learning applications in spam filters, fraud detection, Web searching, credit scoring, advertising placement, and drug design.
Machine Learning as a Service platforms are continuing to emerge and develop, as are improved monitoring and reporting technologies being integrated into an evolving range of smart devices with Internet of Things connectivity.
And with machine learning being hailed by some as the new driver of innovation in data mining and predictive analytics, there should be exciting times ahead.